Research Lifecycle Guide
Research data lifecycle
Research data has a longer lifespan than the project. The research data lifecycle is a model that illustrates the stages of data management and describes how data flow through a research project from start to finish. Data management refers to the process of deciding and documenting how data will be collected, organized, stored and shared.
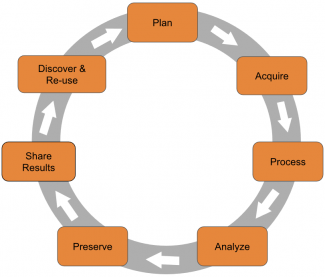
Why manage research data?
This guide is intended to give an overview of best practices for managing research data and to point to additional resources. Some benefits of managing research data are:
- Find and understand data when needed
- Project continuity through researcher or staff changes
- Organized data saves time
- Reduces risk of lost, stolen, or mis-used data
- Comply with funder and journal requirements
- Allows for easier validation of results
- Data can be shared, leading to collaboration and greater impact
In addition, well managed and documented data make it easier to write up research results for publication.
Advantages to planning your research data management practices in advance
Save time: Planning ahead for your data management needs will let you anticipate what you’ll need and organize your data from the start - no last minute scrambling required!
Maintain data integrity: Managing and documenting your data throughout the entire project will allow you and others to understand and more easily use your data in the future.
Meet grant requirements: Many funding agencies require that researchers create and follow a data management and/or data sharing plan.
Advantages to publishing your datasets
Publishing your dataset in an indexed repository that provides a unique, persistent identifier allows it to be:
- More easily discovered
- Cited in its own right, increasing its visibility and impact
- Able to promote the research that created it
More generally, sharing data:
- Can lead to new, unanticipated discoveries
- Provides research material for those with little or no funding
- Promotes innovation and potential new data uses
- Can lead to new collaborations
- Maximizes transparency and accountability
- Encourages improvement and validation of research methods
- Reduces the cost of duplicating data collection